Astronomy Object of the Month: 2022, October
< previous Archive next >
AGNs and the machine learning algorithm
A team of researchers lead by Maria Dainotti, Assistant Professor at the
National Astronomical Observatory of Japan (NAOJ), has achieved a tight
correlation between the redshift values predicted by a machine learning
algorithm and the actual observed redshifts of powerful galaxies called
Active Galactic Nuclei (AGNs). This is the first time that redshifts for
AGNs observed by the Fermi Gamma-Ray Space telescope have been estimated
using machine learning algorithms, and their results offer a fantastic
avenue where the unknown redshifts of many AGNs can be reliably determined.
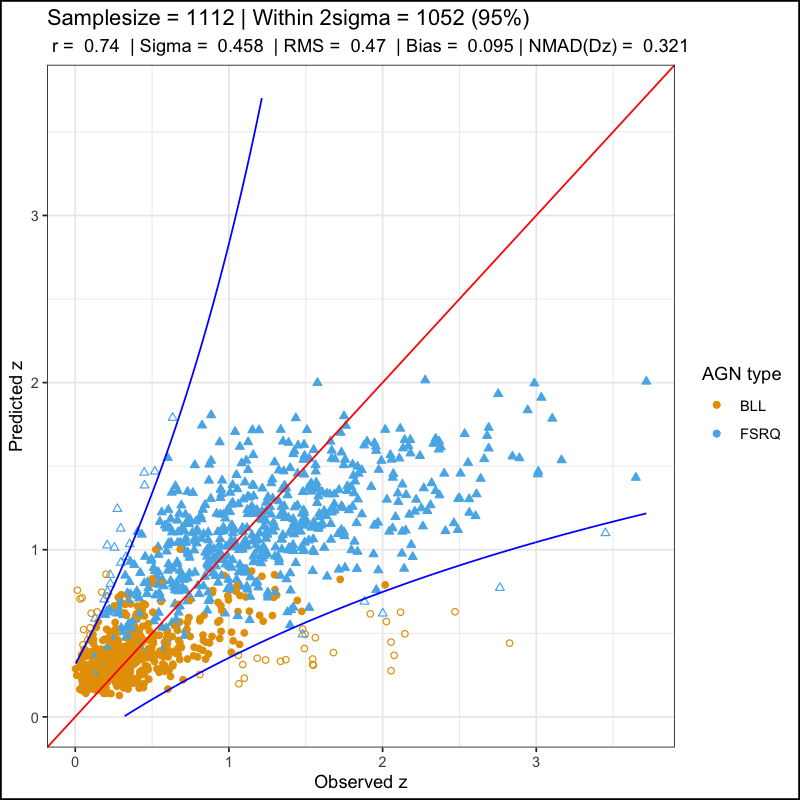
Illustration 1: The correlation
plot showing the observed vs. predicted redshift. Credit: The Authors.
AGNs are some of the most powerful objects in the Universe. Their galactic centers are extremely active regions, emitting huge amounts of radiation. Thus, they are visible across vast distances at different redshifts. However, measuring the redshifts of these galaxies is notoriously difficult as they require extensive spectroscopic observations. This is a difficult and time-consuming task. The problem is even more pronounced for galaxies that are observed primarily in the gamma-ray regime of the electromagnetic spectrum. For example, with the Fermi Gamma-Ray Space Telescope, the state-of-art in gamma-ray observations, only 50% of AGNs have spectroscopic redshift, out of more than 3000 that have been observed so far. This is a significant hurdle for researchers trying to use these AGNs for studying the Universe. A galaxy’s redshift tell us how far away that galaxy is and are hence fundamental for understanding the Universe and the galaxies in it. Thus, there is a requirement for a technique that can estimate the redshift of these galaxies quickly and accurately, without the need for lengthy spectroscopic measurements.
To achieve this goal, the researcher elected to use powerful machine learning algorithms, trained on AGNs from Fermi’s Fourth LAT Catalog (4LAC) that already have redshift measurements. The algorithms learn the underlying correlations between the redshift of a galaxy and its measured gamma-ray properties. Based on these correlations the models try to predict the redshift. As these properties are measured directly by the Fermi telescope, they are easy to obtain. Establishing a strong correlation between them and a galaxy’s redshift can give fast and accurate predictions.
However, an almost limitless choice exists as to which machine learning model should be chosen. Each model has its own advantages and disadvantages, making them more or less suitable for a particular data set. Models that work best for other astronomical data sets may not be effective in the case of Fermi AGNs.
To resolve this puzzle and use the best possible models, the team decided to use a technique called SuperLearner. The SuperLearner belongs to a category of machine learning algorithms called ensemble learning. This means it can combine multiple machine learning models to generate a single model that performs better than any single model. In this way, SuperLearner leverages the strengths and minimizes the weaknesses of multiple machine learning models. This way, the machine learning models best suited for the Fermi data can be chosen and give the best possible results. Armed with this powerful technique, researchers trained six machine learning models on the Fermi data, which were combined inside SuperLearner to produce redshift estimates that have a 74% correlation with the actual observed redshift. This is the highest redshift correlation that has been achieved yet for Fermi AGNs, making this a very important achievement.
The team did not stop there. Using this powerful machine learning model, they predicted the redshift for 305 AGNs from the 4LAC catalog that previously did not have redshift measurements. And as a test of the model’s real work perform, estimated the redshift of 47 AGNs that had not been used for training the model. Here also they achieved a 73% correlation.
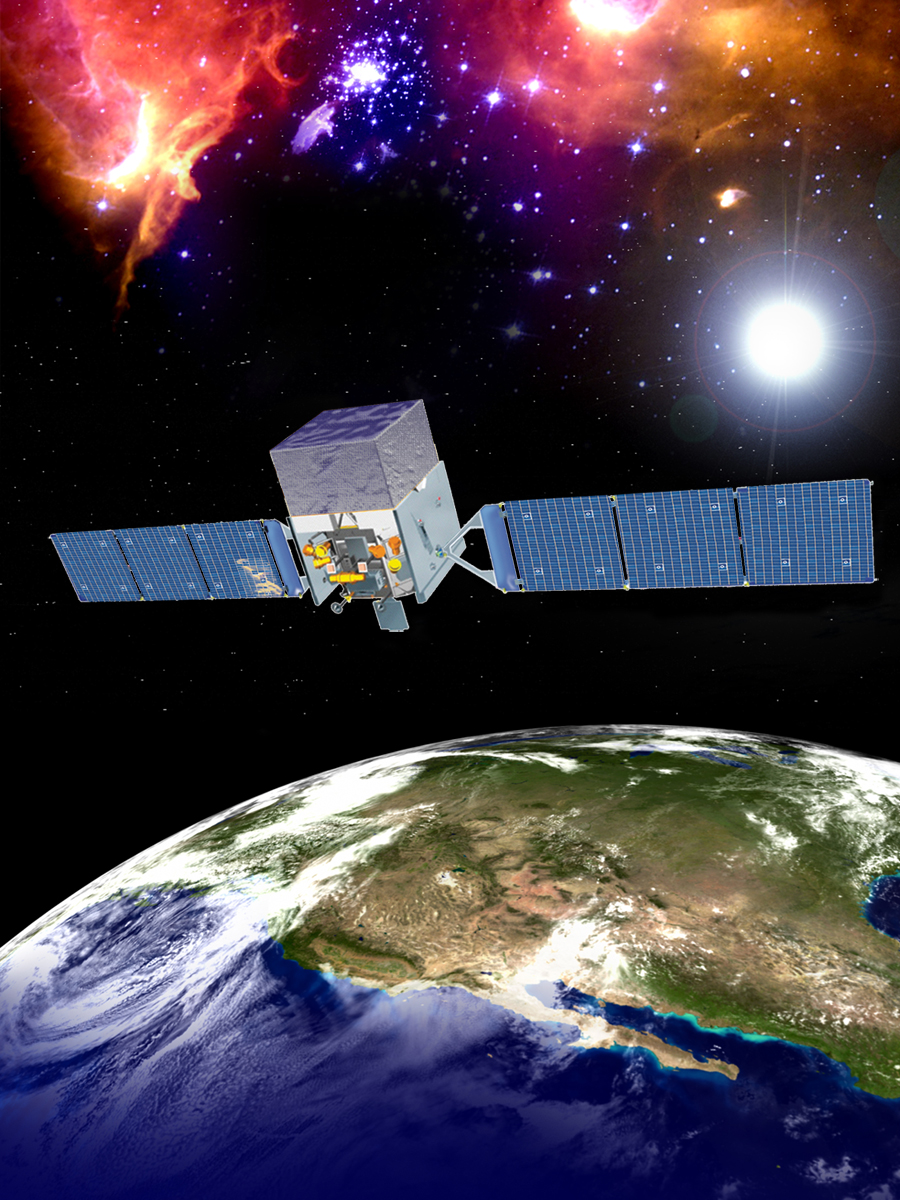
Illustration 2. The Fermi Gamma-ray Space Telescope. Credit: NASA.
These pioneering work have shown that fast and accurate redshift estimates of AGNs are possible using their observed gamma-ray properties. They also show that the missing values present in the 4LAC and other similar catalogs can be imputed so that nearly complete observations can also be used in the training of such models. As of this writing, the three articles published by the group are the only ones exploring the redshift estimation of gamma-ray loud AGNs of the 4LAC.
Original publication: These results appeared first is Dainotti et al., Predicting the Redshift of γ-Ray-loud AGNs Using Supervised Machine Learning, the Astrophysical Journal, 2021, followed by Narendra et al., Predicting the Redshift of Gamma-Ray Loud AGNs Using Supervised Machine Learning. II (the Astrophysical Journal 2022) and finally in Gibson et al., Using Multivariate Imputation by Chained Equations to Predict Redshifts of Active Galactic Nuclei, in Frontiers in Astronomy and Space Sciences on March 4, 2022.
The research was conducted at the Department of Stellar and Extragalactic Astronomy of the Jagiellonian University’s Astronomical Observatory (OA UJ). This research was supported by the Polish National Science Centre grant UMO-2018/30/M/ST9/00757 and by Polish Ministry of Science and Higher Education grant DIR/WK/2018/12.
Aditya Narendra Astronomical Observatory Jagiellonian University A.Narendra [@] oa.uj.edu.pl |